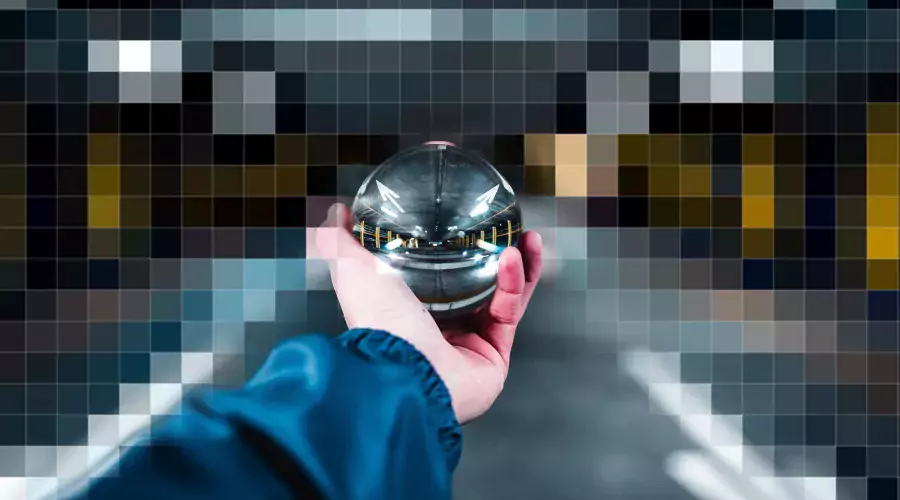
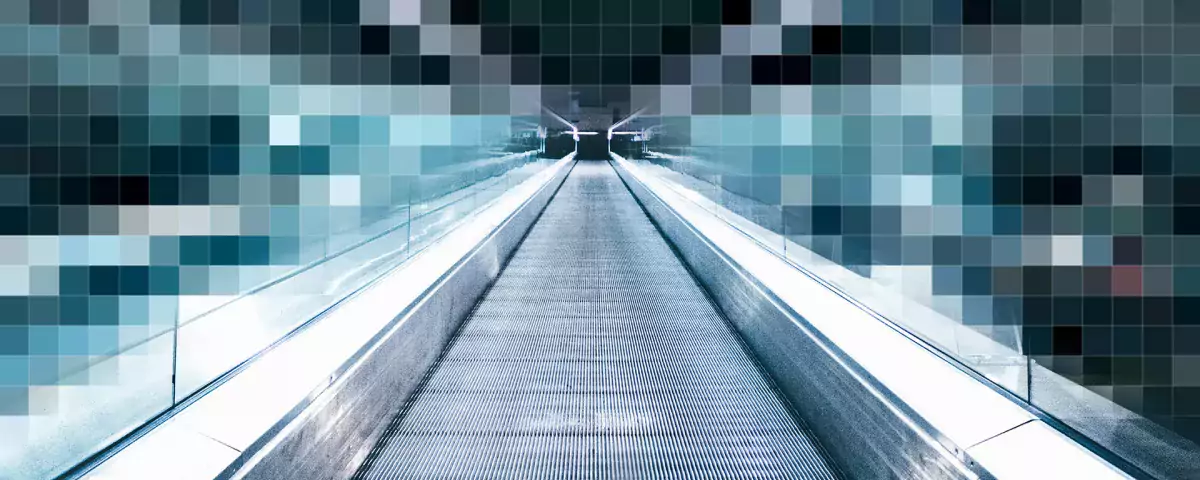
24.04.2024 | News Generative AI needs a plan
Generative AI holds enormous potential for organizations in all sectors. It is therefore no wonder that stakeholders are wasting no time and want to get started as quickly as possible. However, an ill-conceived "just go for it" approach is not a good idea, as generative AI risks becoming an expensive, pointless, and possibly even dangerous adventure. IntraFind lists five questions that those responsible should ask themselves before investing in GenAI projects.
1. What do we want to achieve? First, companies need to have a clear idea, which specific benefits they want to achieve with generative AI. They should therefore identify, define, prioritize and – above all – test use cases to derive an overall strategy from the experience gained. The possible use cases for GenAI are by no means limited to the popular chatbot applications. Generative AI can also summarize information from different and extensive documents, be used as a digital assistant in first-level customer support or extract certain data from files.
2. Which AI model is appropriate? The right AI language model should be selected depending on the use case. In addition to the well-known proprietary models such as GPT from Open AI or Luminous from the German provider Aleph Alpha, there are other powerful solutions from the open-source community. The language models also differ in terms of model size and the so-called context size, which determines how much text a model can view at once. These factors have an impact on the quality, costs, and performance of a GenAI application.
3. Which operating model should we use? An important aspect of the implementation of generative AI is data protection. In sensitive government environments or when GenAI applications process intellectual property or personal information in accordance with the GDPR, it may be necessary to operate these applications on-premises for compliance reasons. In this case, companies have the protection of their data under their own control and avoid the risk of vendor lock-in but must set up the necessary GPU infrastructure themselves. With the SaaS operating model, it should be noted that the costs are currently still difficult to calculate. Many large SaaS providers currently charge according to a complicated "token-based" procedure that is based on the number of words in the questions and answers.
4. How do we integrate our data? Most use cases require language models to be used with their own data. One way to do this is to train the models with this data. However, there is still the inherent risk of hallucinations. In addition, the models must be regularly and expensively retrained to remain up to date. Retrieval Augmented Generation (RAG) offers an alternative option by combining generative AI with enterprise search. This involves integrating the enterprise search retrieval systems, which search an organization's own data for relevant information in response to search queries. The found documents are then passed to the language model to generate the answer. As a result, the answers are always based on up-to-date information and the risk of hallucinations is significantly reduced. Enterprise Search also automatically considers access rights to company data. Employees or customers only receive information in their answers they are authorized to access and view. This central security aspect is often overlooked in the general GenAI euphoria.
5 How do we break down data silos? If GenAI applications only have incomplete data available, they produce incomplete and incorrect output. Organizations must therefore find a way to make data silos usable. Enterprise search software is a good way to do this. It can connect a wide variety of data sources via connectors, regardless of whether they are structured or unstructured data, and bring them together in one central search index. This provides a holistic and valid database for GenAI applications, which can be searched by the enterprise search software as part of a RAG architecture.
"Organizations need a GenAI strategy," explains IntraFind CEO Franz Kögl. "They know their processes best themselves and are therefore best placed to identify the use cases that will benefit them most. Experienced experts and service providers can then help them select suitable AI models and operating options, integrate their data, and implement tailor-made solutions.
Related Articles
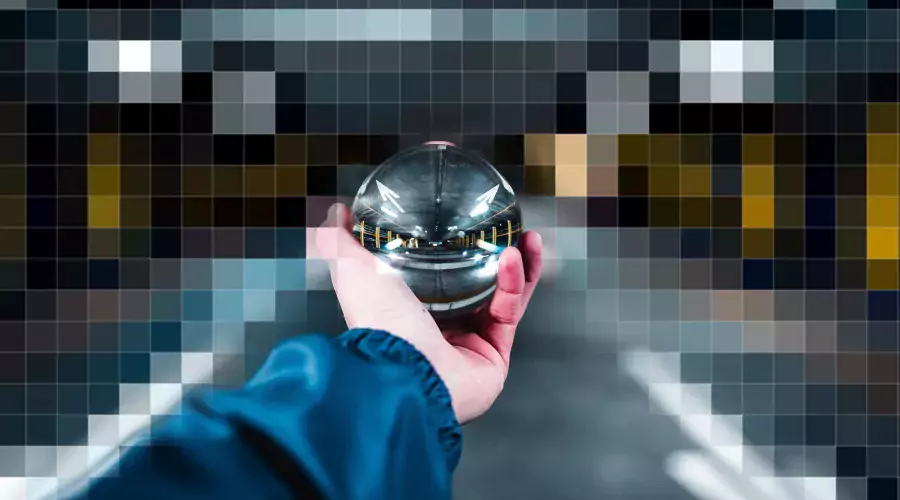
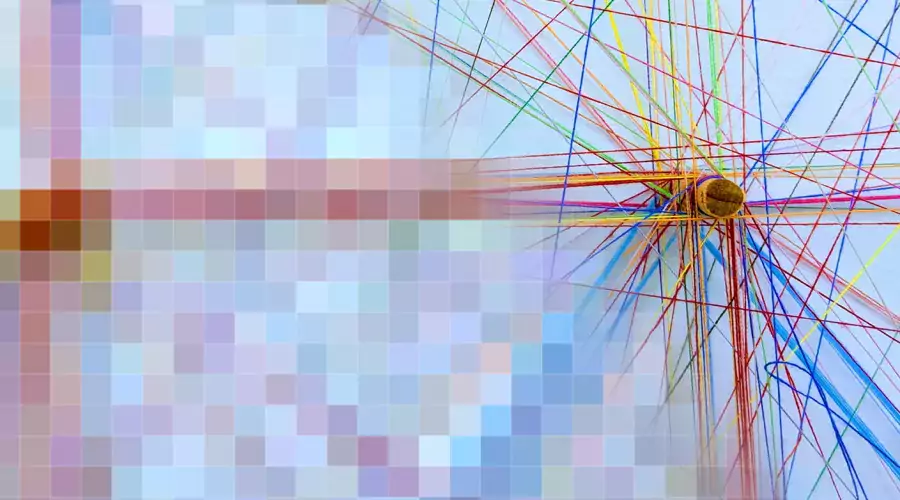
Generative AI: From hype to practical application in business
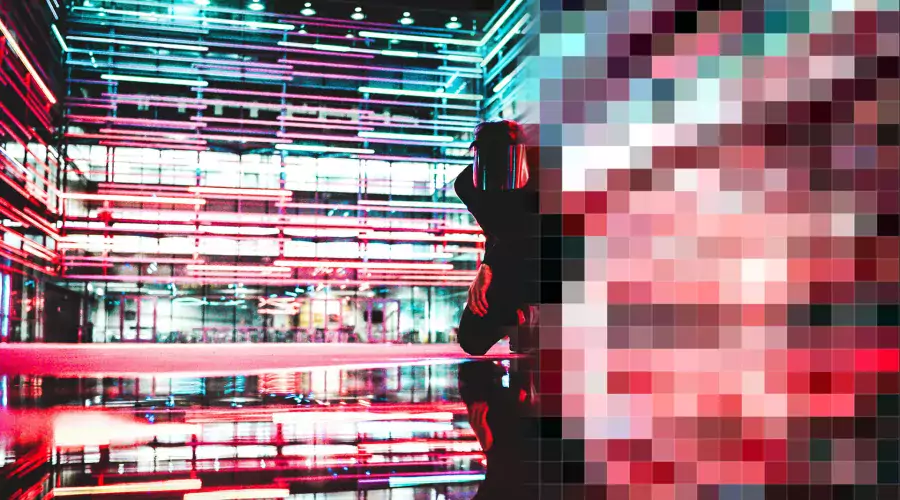